Rechercher dans ce blog
Friday, July 31, 2020
Massive Samsung sale at Best Buy discounts Chromebooks, TVs, and more - Tom's Guide
Just in time for the weekend, Best Buy is having a massive Samsung sale with discounts on Samsung TVs, Galaxy smartphones, tablets, and more. The 3-day Samsung sale also includes Samsung appliances like ovens, washers, and refrigerators.
The 3-day Samsung sale at Best Buy also packs many excellent back to school sales with discounts on Samsung Chromebooks and monitors. We're rounding up the best deals below, just remember that the sale is valid through Sunday only.
Samsung 24" LCD: was $149 now $109 @ Best Buy
The Samsung SF350 is a 24-inch 1080p monitor that's good for both work and play. It sports a 4ms response time, which is great for gaming and it has four brightness modes that optimize the monitor's display for everything from gaming to working with spreadsheets. It has HDMI and VGA inputs. View Deal
Samsung Galaxy Buds Plus: was $149 now $129 @ Best Buy
The Samsung Galaxy Buds are a great AirPods alternative for Android users. They offer seamless device pairing, water/sweat resistance, and long battery life. They're now on sale for $129.99 at Best Buy. View Deal
Samsung tablet sale: from $129 @ Best Buy
Best Buy is having an epic sale on all things Samsung and as part of its 3-day sale it's slashing the price of Galaxy tablets. For instance, you can get the Galaxy Tab S6 Lite for $319.99 ($30 off). Even better, it comes with a $20 Best Buy gift card. View Deal
Galaxy Chromebook 4K: was $999 now $799 @ Best Buy
The Samsung Galaxy Chromebook is one of the few Chromebooks to pack a 13.3-inch 4K screen. It also packs a Core i5-i5-10210U CPU, 8GB of RAM, and a 256GB SSD. The 2-in-one cuts no corners and offers excellent performance. View Deal
August 01, 2020 at 04:09AM
https://ift.tt/2EAWA4J
Massive Samsung sale at Best Buy discounts Chromebooks, TVs, and more - Tom's Guide
https://ift.tt/2O3clnm
Android Circuit: New Galaxy S21 Specs, Three Nokia Surprises, Samsung Loses To Huawei - Forbes
Taking a look back at seven days of news and headlines across the world of Android, this week’s Android Circuit includes Galaxy S21 leaks, Huawei beating Samsung, OnePlus Nord reviews, Motorola’s One Fusion Plus, three new Nokia handsets, Surface Duo heads to Canada, and CES goes online only…
Android Circuit is here to remind you of a few of the many things that have happened around Android in the last week (and you can find the weekly Apple news digest here).
The Surprise Inside The Next Galaxy S
With the Galaxy Note 20 family set to launch during August, attention will be turning towards the flagship Galaxy S21 release in early 2021. And with the first benchmark results already leaked, there’s a surprisingly powerful change planned. Forbes’ Gordon Kelly:
"Thanks to Samsung’s own leaked benchmarks, we know the chipset inside the Galaxy S21 is potentially transformative. Based on Samsung’s own roadmap, this is understood to be a Exynos 1000 made in conjunction with AMD using a custom Radeon GPU. And those benchmarks? Gaming performance delivered up to 2.5x the performance of a Snapdragon 865. That’s a multi-generational improvement and means it not only leaves every rival Android smartphone eating its dust, but every iPhone as well."
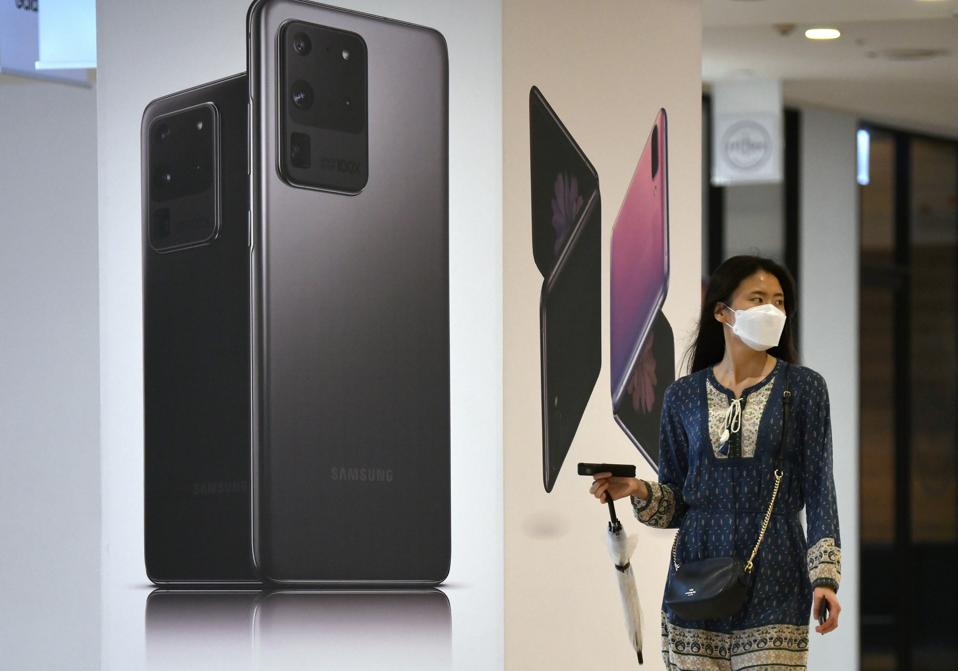
A woman walks past an advertisement for the Samsung Galaxy S20 Ultra and Galaxy Z Flip smartphones ... [+] at a showroom in Seoul on July 30, 2020. - Samsung Electronics reported on July 30 its net profit grew 7.3 percent year-on-year in the second quarter, with strong demand for memory chips overcoming the impact of the coronavirus pandemic on smartphone sales. (Photo by Jung Yeon-je / AFP) (Photo by JUNG YEON-JE/AFP via Getty Images)
AFP via Getty ImagesHuawei Tops Samsung In Worldwide Sales
Samsung has lost its top spot in the worldwide smartphone market, according to Canalys’ numbers for Q2. Even with its inclusion on the US Entity list and overseas sales dropping by 27 percent, the Chinese-based manufacturers 55.8 million sales was enough for the number one award. From the report:
"It marks the first quarter in nine years that a company other than Samsung or Apple has led the market. Huawei shipped 55.8 million devices, down 5% year on year. But second-placed Samsung shipped 53.7 million smartphones, a 30% fall against Q2 2019.
Huawei is still subject to US government restrictions, which have stifled its business outside of mainland China. Its overseas shipments fell 27% in Q2. But it has grown to dominate its domestic market, boosting its Chinese shipments by 8% in Q2, and it now sells over 70% of its smartphones in mainland China. China has emerged strongest from the coronavirus pandemic, with factories reopened, economic development continuing and tight controls on new outbreaks.”
OnePlus Nord Reviewed
This week saw the OnePlus Nord get the full review treatment from the tech community. Many commented on the aggressive pricing of the mid-range handset, the fast-refreshing screen, and the weaker than expected plastic chassis. Ron Amadeo’s review covers all the bases:
For a mid-range phone, there really isn't much missing here. You still get NFC, the same in-screen optical fingerprint reader as every other phone, and OnePlus' fantastic 30W quick charging. Compared to a flagship phone, the big list of missing features would be the aforementioned single speaker instead of stereo, no wireless charging, and no official water-resistance rating. OnePlus says the phone still has gaskets to provide some water resistance, but with no official rating it's hard to say how much, like "is this submersible?"
I’ve taken a closer look at the Nord as well, read my full review here. Currently scheduled for a full release in Europe and India (apart from a special 50 handset run), many OnePlus followers have been asking for a US handsets. XDA Developers discusses a SnapDragon 690 powered Nord handset for the North American markets:
“…we suspect they’ll be part of the OnePlus Nord brand. OnePlus has already confirmed that a future Nord-branded device is coming to the U.S. later this year, and it’s likely that future Nord devices will launch in Europe and India, too. If the upcoming device(s) are indeed powered by the Qualcomm Snapdragon 690 mobile platform, then they’ll cost even less than the current OnePlus Nord, which currently starts at £379/€399/₹27,999 in the UK/Europe/India.”
More on the next Nord can be read here.

OnePlus Nord (Ewan Spence)
Ewan SpenceMotorola’s Mid-Range Mobile Pitch
Staying with the mid-range market (it’s getting awfully crowded, isn’t it), Motorola’s One Fusion+ brings together a balance of features to create another handset with some big headline features (the quad camera, the 6.55 inch notchless screen, and a powerful processor) while making compromises in other areas (there’s no fast charging, and you have an LCD panel rather than OLED). Tushar Mehta reviews the handset, including the benefits of the huge battery:
"The Motorola One Fusion+ features a massive 5000mAh battery. While the battery is one of the culprits behind the phone’s heaviness, it allows for long-lasting battery life. The massive battery easily powers the phone for two days with basic usage and over a day with demanding tasks including streaming online videos continuously and gaming for hours.
"…The battery life of the Motorola One Fusion+ is incredible. It is in fact on par with the Redmi Note 9 Pro (Note 9S) we reviewed in March earlier this year. What’s better about the Motorola One Fusion+ is that it provides similar battery backup without aggressively killing background apps and activities.”
Read the full review at XDA Developers.
Three Wise Nokias For Christmas
A triple header of Nokia branded handsets from HMD Global are expected to launch this year.. The ‘mark 3’ variants of the Nokia 6, Nokia 7 and Nokia 9 PureView should be with us by the end of 2020. Nayan Mishra reports:
"Sources indicate that HMD is going ahead with the testing of Nokia 6.3, Nokia 7.3, and Nokia 9.3 prototypes and it is progressing fine. We recently reported about two Nokia 7.3 prototypes in testing and that one of them has 5G support indicating that HMD may be planning to bring 5G to even lower price bracket.”
More details on the handsets at Nokia Power User.
Surface Duo Heads To Canada
Meanwhile, Microsoft’s Android-powered Surface Duo has picked up another government certification. It looks like the dual-screened device is heading to Canada. Mayank Parmar reports:
"Microsoft Surface Duo with model number 1930 was recently discovered on the FCC website and it also passed Bluetooth SIG certification… Notably, another variant of the Surface Duo has appeared in Canada-based UL certifications and the model number is “1930r”, which could be for a high-end variant of the device or its locked/unlocked version.”
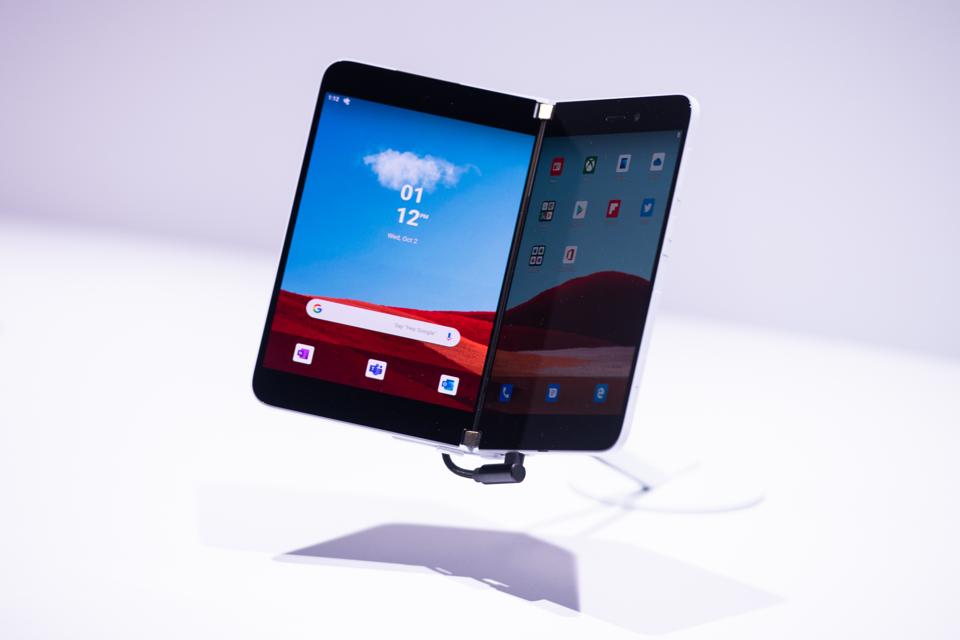
The Microsoft Corp. Surface Duo smartphone is displayed during a product event in New York, U.S., on ... [+] Wednesday, Oct. 2, 2019. Microsoft unveiled a dual-screen, foldable phone that will run on Google's Android operating system, jumping back into a market it exited years ago. Photographer: Mark Kauzlarich/Bloomberg
© 2019 Bloomberg Finance LPAnd Finally…
One of the key technology events in the annual tech calendar is CES. Any other year and the full circus would be booking hotel rooms, halls, and conference spaces in Las Vegas. 2021 is not going to be like any other year. Due to the ongoing coronavirus pandemic, Consumer Technology Association - who run CES - has made the decision that 2021’s CES will be “All Digital”. From the press release:
"Amid the pandemic and growing global health concerns about the spread of COVID-19, it's just not possible to safely convene tens of thousands of people in Las Vegas in early January 2021 to meet and do business in person," said Gary Shapiro, president and CEO, CTA.
"Technology helps us all work, learn and connect during the pandemic – and that innovation will also help us reimagine CES 2021 and bring together the tech community in a meaningful way. By shifting to an all-digital platform for 2021, we can deliver a unique experience that helps our exhibitors connect with existing and new audiences”
Android Circuit rounds up the news from the Android world every weekend here on Forbes. Don’t forget to follow me so you don’t miss any coverage in the future, and of course read the sister column in Apple Loop! Last week’s Android Circuit can be found here, and if you have any news and links you’d like to see featured in Android Circuit, get in touch!
August 01, 2020 at 06:05AM
https://ift.tt/33dxmni
Android Circuit: New Galaxy S21 Specs, Three Nokia Surprises, Samsung Loses To Huawei - Forbes
https://ift.tt/2O3clnm
Did Huawei Just Beat Trump’s Blacklist? - Forbes

LightRocket via Getty Images
Context, as they say, is everything. Reading headlines this week that lauded Huawei for “overtaking,” even “eclipsing,” Samsung to become the world number one for smartphone sales, “despite U.S. sanctions,” you’d think Trump’s blacklist had derailed. Seizing Samsung’s crown has been Huawei ambition all along—and the company had seemed on course before the blacklist hit last May.
Since the blacklist hit, though, it’s been a very different story. The loss of Google from new phones has seen them fall flat in key international markets. Huawei has been accelerating efforts to build a third-way, a new version of Android’s ecosystem that it has badged Huawei Mobile Services, and which is intended to break the Google lock on its users. To do so is a huge challenge.
And so, unsurprisingly, Huawei was quick to celebrate becoming the world’s number one for the first time, “demonstrating exceptional resilience in difficult times,” it said. Does this success mean Huawei “doesn't need Google's help to succeed,” as one tech site suggested? Does this really represent a major setback for the Trump administration’s campaign against the company?
No. Absolutely not. In reality, nothing has changed. Huawei securing the top spot for the second quarter came as a result of three things—none of which involved it beating the blacklist. First, Huawei recorded staggering sales success in its home Chinese market—increasing its market share from 33% during the same quarter last year to 46% this time around—all at the expense of local rivals.
Second, China was first into a COVID-19 lockdown and so was the first major market to come out of it. This resulted in a recovery in smartphone sales in Huawei’s strongest market, while Samsung’s key markets—the U.S., for example—lagged behind. The sheer scale of Huawei dominance in China, the world’s largest smartphone market, was enough to influence the global rankings.
And, third, Huawei managed to extend the life of its pre-blacklist phones through a series of facelifts, adding sales in European markets where newer flagships absent Google were falling flat. And while this had a modest impact given 70% of Huawei’s phone sales were in China, the difference between Huawei and Samsung was marginal—55.8 to 54.2 million or 55.8 to 53.7 million, depending on the report, and so every little helped.
For Huawei, 2020 was always set to be a torrid year. The company warned as much in its New Year message to staff, and nothing that has happened since has suggested those warnings were wrong. Smartphone sales had become the leading driver of Huawei’s growth and profitability before the blacklist, and now that’s narrowed further to phone sales in China. This is a serious over-exposure, and it won’t reduce any time soon.
Until May, the issue for Huawei’s smartphone sales—outside China, where Google is banned—was that loss of U.S. software and services. But the blacklist was extended in May, on its first anniversary, and now Huawei is facing restrictions on the cutting-edge chips inside its flagships as well. Beyond smartphones, this has hit Huawei hard in the 5G equipment market. Here Chinese consumers can’t make up the difference. And while Huawei is guaranteed the lion’s share of 5G network kit sales in China, it is losing deals elsewhere—most notably in the U.K. All down to that U.S. blacklist.
The smartphone ranking news is significant, “marking the first quarter in nine years that a company other than Samsung or Apple has led the market,” but it is irrelevant as regards U.S. sanctions—it tells us nothing. The real news is that Huawei’s dominance in China and Samsung’s coronavirus-related sales lag elsewhere have coincided. And so the results for the balance of the year will be more down to economic recoveries as anything Huawei or Samsung can do.
August 01, 2020 at 05:21AM
https://ift.tt/3hY6FHc
Did Huawei Just Beat Trump’s Blacklist? - Forbes
https://ift.tt/2NcqjCg
Witnesses to prove Huawei’s Meng Wanzhou lied, supporting her extradition: docs - Globalnews.ca
Documents from lawyers for Canada’s attorney general say a series of witnesses will prove Huawei executive Meng Wanzhou lied to HSBC bank about the company’s relationship’s with Skycom in Iran.
The documents released Friday are the government’s arguments to be used during a hearing next April and they say there’s enough to prove fraud in support of Meng’s extradition to the United States.
The documents say witnesses, including former employees of Huawei, FBI investigators and officials with HSBC — the bank at the centre of the allegations — will say Meng falsely said Huawei didn’t control Skycom.
Meng was arrested at Vancouver’s airport on a request from the United States over allegations both she and Huawei broke American sanctions against Iran, accusations both have denied.
Story continues below advertisement

The documents say witnesses will tell the court Meng reassured a senior HSBC executive that Skycom was a local partner of Huawei’s and that the Chinese company had divested any shares in the company in Iran.
Hours after that meeting, Huawei announced it had received a $1.5-billion loan from a group of international banks with HSBC was the principal lender.
A few days later, the unnamed witness emailed other senior HSBC personnel, “stating that ‘Everything appears to be above board,’ ‘Huawei has stated that it complies with all laws and sanctions,’ and ‘I’m pretty much reassured,'” the documents say.
Trending Stories
The HSBC risk committee responsible for the Asia-Pacific region met in Hong Kong and considered that Huawei advised HSBC that its shares in Skycom were sold in 2009 and Meng resigned her position on the board in the same year, the documents say.
Story continues below advertisement
“The evidence demonstrates that Ms. Meng deliberately made dishonest representations to HSBC in an attempt to preserve Huawei’s relationship with the bank, knowing that in so doing, HSBC would be exposed to risk of economic loss.”

The evidence establishes fraud and is enough to extradite Meng to the United States, the documents say.
Meng’s arrest has generated increasing friction between Canada and China. China’s arrests of Canadians Michael Kovrig and Michael Spavor and subsequent allegations of spying are widely seen as attempts by China to pressure Canada to release Meng.
Her lawyers have accused U.S. President Donald Trump of poisoning the extradition case against Meng with his interference and attempt to use her as a “bargaining chip” in the trade dispute between the U.S. and China.

The documents released Friday will be used during the committal hearing planned for April 2021.
Story continues below advertisement
Before that, there will be other legal arguments, including a hearing next February where Meng’s lawyers will argue that she was subject to an abuse of process during her arrest in December 2018.
© 2020 The Canadian Press
August 01, 2020 at 03:43AM
https://ift.tt/33cA8cn
Witnesses to prove Huawei’s Meng Wanzhou lied, supporting her extradition: docs - Globalnews.ca
https://ift.tt/2NcqjCg
The field of natural language processing is chasing the wrong goal - MIT Technology Review
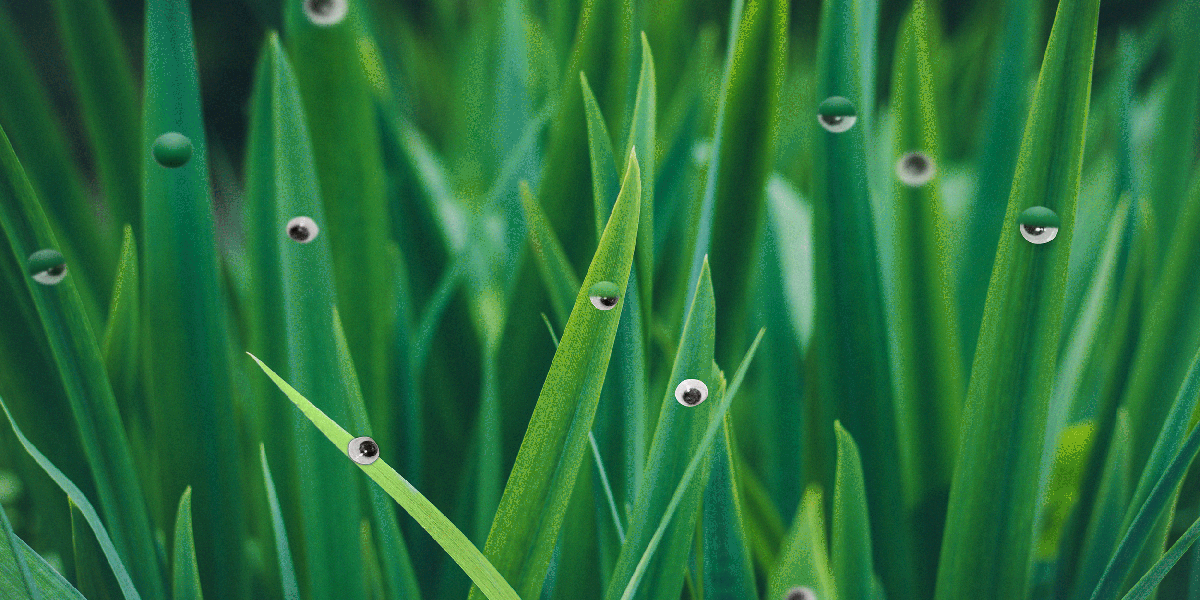
At a typical annual meeting of the Association for Computational Linguistics (ACL), the program is a parade of titles like “A Structured Variational Autoencoder for Contextual Morphological Inflection.” The same technical flavor permeates the papers, the research talks, and many hallway chats.
At this year’s conference in July, though, something felt different—and it wasn’t just the virtual format. Attendees’ conversations were unusually introspective about the core methods and objectives of natural-language processing (NLP), the branch of AI focused on creating systems that analyze or generate human language. Papers in this year’s new “Theme” track asked questions like: Are current methods really enough to achieve the field’s ultimate goals? What even are those goals?
My colleagues and I at Elemental Cognition, an AI research firm based in Connecticut and New York, see the angst as justified. In fact, we believe that the field needs a transformation, not just in system design, but in a less glamorous area: evaluation.
The current NLP zeitgeist arose from half a decade of steady improvements under the standard evaluation paradigm. Systems’ ability to comprehend has generally been measured on benchmark data sets consisting of thousands of questions, each accompanied by passages containing the answer. When deep neural networks swept the field in the mid-2010s, they brought a quantum leap in performance. Subsequent rounds of work kept inching scores ever closer to 100% (or at least to parity with humans).
So researchers would publish new data sets of even trickier questions, only to see even bigger neural networks quickly post impressive scores. Much of today’s reading comprehension research entails carefully tweaking models to eke out a few more percentage points on the latest data sets. “State of the art” has practically become a proper noun: “We beat SOTA on SQuAD by 2.4 points!”
But many people in the field are growing weary of such leaderboard-chasing. What has the world really gained if a massive neural network achieves SOTA on some benchmark by a point or two? It’s not as though anyone cares about answering these questions for their own sake; winning the leaderboard is an academic exercise that may not make real-world tools any better. Indeed, many apparent improvements emerge not from general comprehension abilities, but from models’ extraordinary skill at exploiting spurious patterns in the data. Do recent “advances” really translate into helping people solve problems?
Such doubts are more than abstract fretting; whether systems are truly proficient at language comprehension has real stakes for society. Of course, “comprehension” entails a broad collection of skills. For simpler applications—such as retrieving Wikipedia factoids or assessing the sentiment in product reviews—modern methods do pretty well. But when people imagine computers that comprehend language, they envision far more sophisticated behaviors: legal tools that help people analyze their predicaments; research assistants that synthesize information from across the web; robots or game characters that carry out detailed instructions.
Today’s models are nowhere close to achieving that level of comprehension—and it’s not clear that yet another SOTA paper will bring the field any closer.
How did the NLP community end up with such a gap between on-paper evaluations and real-world ability? In an ACL position paper, my colleagues and I argue that in the quest to reach difficult benchmarks, evaluations have lost sight of the real targets: those sophisticated downstream applications. To borrow a line from the paper, the NLP researchers have been training to become professional sprinters by “glancing around the gym and adopting any exercises that look hard.”
To bring evaluations more in line with the targets, it helps to consider what holds today’s systems back.
A human reading a passage will build a detailed representation of entities, locations, events, and their relationships—a “mental model” of the world described in the text. The reader can then fill in missing details in the model, extrapolate a scene forward or backward, or even hypothesize about counterfactual alternatives.
This sort of modeling and reasoning is precisely what automated research assistants or game characters must do—and it’s conspicuously missing from today’s systems. An NLP researcher can usually stump a state-of-the-art reading comprehension system within a few tries. One reliable technique is to probe the system’s model of the world, which can leave even the much-ballyhooed GPT-3 babbling about cycloptic blades of grass.
Imbuing automated readers with world models will require major innovations in system design, as discussed in several Theme-track submissions. But our argument is more basic: however systems are implemented, if they need to have faithful world models, then evaluations should systematically test whether they have faithful world models.
Stated so baldly, that may sound obvious, but it’s rarely done. Research groups like the Allen Institute for AI have proposed other ways to harden the evaluations, such as targeting diverse linguistic structures, asking questions that rely on multiple reasoning steps, or even just aggregating many benchmarks. Other researchers, such as Yejin Choi’s group at the University of Washington, have focused on testing common sense, which pulls in aspects of a world model. Such efforts are helpful, but they generally still focus on compiling questions that today’s systems struggle to answer.
We’re proposing a more fundamental shift: to construct more meaningful evaluations, NLP researchers should start by thoroughly specifying what a system’s world model should contain to be useful for downstream applications. We call such an account a “template of understanding.”
One particularly promising testbed for this approach is fictional stories. Original stories are information-rich, un-Googleable, and central to many applications, making them an ideal test of reading comprehension skills. Drawing on cognitive science literature about human readers, our CEO David Ferrucci has proposed a four-part template for testing an AI system’s ability to understand stories.
- Spatial: Where is everything located and how is it positioned throughout the story?
- Temporal: What events occur and when?
- Causal: How do events lead mechanistically to other events?
- Motivational: Why do the characters decide to take the actions they take?
By systematically asking these questions about all the entities and events in a story, NLP researchers can score systems’ comprehension in a principled way, probing for the world models that systems actually need.
It’s heartening to see the NLP community reflect on what’s missing from today’s technologies. We hope this thinking will lead to substantial investment not just in new algorithms, but in new and more rigorous ways of measuring machines’ comprehension. Such work may not make as many headlines, but we suspect that investment in this area will push the field forward at least as much as the next gargantuan model.
Jesse Dunietz is a researcher at Elemental Cognition, where he works on developing rigorous evaluations for reading comprehension systems. He is also an educational designer for MIT’s Communication Lab and a science writer.
July 31, 2020 at 09:45PM
https://ift.tt/39LgrJU
The field of natural language processing is chasing the wrong goal - MIT Technology Review
https://ift.tt/2APQ0pp
The 6 unholy AI systems thou shalt not develop - The Next Web
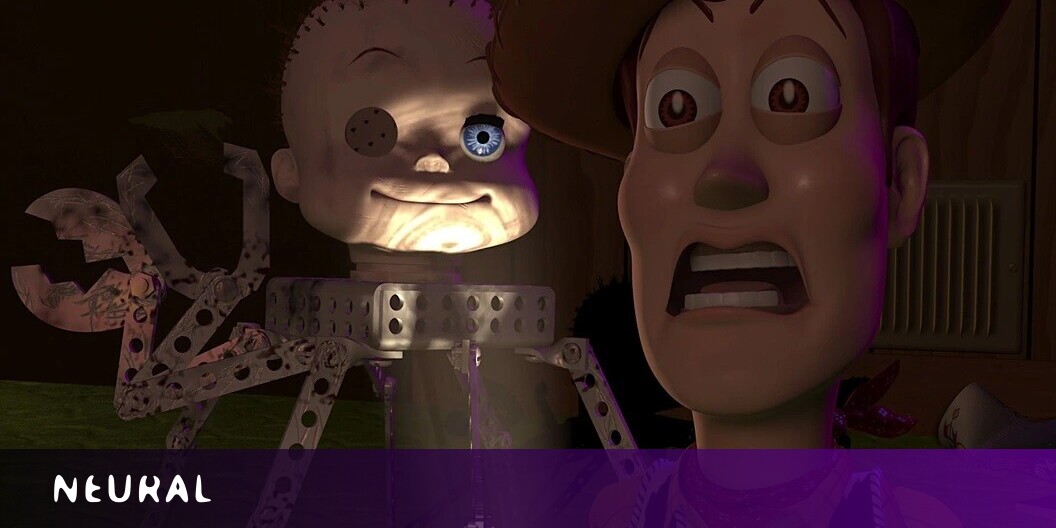
Artificial intelligence may be the most powerful tool humans have. When applied properly to a problem suited for it, AI allows humans to do amazing things. We can diagnose cancer at a glance or give a voice to those who cannot speak by simply applying the right algorithm in the correct way.
But AI isn’t a panacea or cure-all. In fact, when improperly applied, it’s a dangerous snake oil that should be avoided at all costs. To that end, I present six types of AI that I believe ethical developers should avoid.
First though, a brief explanation. I’m not passing judgment on developer intent or debating the core reasoning behind the development of these systems, but instead recognizing six areas where AI cannot provide a benefit to humans and is likely to harm us.
I’m not including military technology like autonomous weapons or AI-powered targeting systems because we do need debate on those technologies. And we’ve also intentionally left “knife technologies” off of this list. Those are techs such as DeepFakes which can arguably be used for good and evil, much like a knife can be used to chop vegetables or stab people.
Instead, I’ve focused on those technologies that distort the very problem they’re purported to solve. We’ll begin with the low hanging fruits: criminality and punishment.
Criminality
AI cannot determine the likelihood that a given individual, group of people, or specific population will commit a crime. Neither humans nor machines are psychic.
[Related: Predictive policing is a bigger scam than psychic detectives]
Predictive policing is racist. It uses historical data to predict where crime is most likely to occur based on past trends. If police visit a specific neighborhood more often than others and arrest people in that neighborhood regularly, an AI trained on data from that geographic area will determine that crime is more likely to happen in that neighborhood than others.
Put in another perspective: If you shop at Wal Mart exclusively for toilet paper and you’ve never purchased toilet paper from Amazon, you’re more likely to associate toilet paper with Wal Mart than Amazon. That doesn’t mean there’s more toilet paper at Wal Mart.
AI that attempts to predict criminality is fundamentally flawed because the vast majority of crimes go unnoticed. Developers are basically creating machines that validate whatever the cops have already done. They don’t predict crime, they just reinforce the false idea that over-policing low-income neighborhoods lowers crime. This makes the police look good.
But it doesn’t actually indicate which individuals in a society are likely to commit a crime. In fact, at best it just keeps an eye on those that’ve already been caught. At worst, these systems are a criminal’s best friend. The more they’re used, the more likely crime will perpetuate in areas where police presence is traditionally low.
Punishment
Algorithms cannot determine how likely a human is to commit a crime again after being convicted of a previous crime. See above, psychics do not exist. What a machine can do is take historical sentencing records and come to the mathematically sensible solution that people who are punished harshest tend to be the most recidivist and, thus, falsely indicate that Black people must be more likely to commit crimes than white people.
This is exactly what happens when developers use the wrong data for a problem. If you’re supposed to add 2 + 2, there’s no use for an apple in your equation. In this case, what that means is historical data on people who’ve committed crimes after release from the judicial system isn’t relevant to whether or not any specific individual will follow suit.
[Read: Why the criminal justice system should abandon algorithms]
People aren’t motivated to commit crimes because strangers they’ve never met were motivated to commit crimes upon release from custody. This information, how the general populace responds to release from incarceration, is useful for determining whether our justice system is actually rehabilitating people or not, but it cannot determine how likely a “Black male, 32, Boston, first offense” is to commit a post-conviction crime.
No amount of data can actually predict whether a human will commit a crime. It’s important to understand this because you can’t un-arrest, un-incarcerate, or un-traumatize a person who has been wrongfully arrested, imprisoned, or sentenced based on erroneous evidence generated from an algorithm.
Gender
Here’s a fun one. A company recently developed an algorithm that could allegedly determine someone’s gender from their name, email address, or social media handle. Sure, and I’ve got an algorithm that makes your poop smell like watermelon Jolly Ranchers (note: I do not. That’s sarcasm. Don’t email me.).
AI cannot determine a person’s gender from anything other than that person’s explicit description of their gender. Why? You’ll see a theme developing here: because psychics don’t exist.
Humans cannot look at other humans and determine their gender. We can guess, and we’re often correct, but let’s do a quick thought experiment:
If you lined up every human on the planet and looked at their faces to determine whether they were “male” or “female” how many would you get wrong? Do you think an AI is better at determining human gender in the margin cases where even you, a person who can read and everything, can’t get it right? Can you tell an intersex person by their face? Can you always tell what gender someone was assigned at birth by looking at their face? What if they’re Black or Asian?
Let’s simplify: even if your PhD is in gender studies and you’ve studied AI under Ian Goodfellow, you cannot build a machine that understands gender at a glance because humans themselves do not. You cannot tell every person’s gender, which means your machine will get some wrong. There are no domains where misgendering humans is beneficial, but there are myriad domains where doing so will cause direct harm to the humans who have been misgendered.
Any tool that attempts to predict human gender based on extraneous data has no use other than as a weapon against the transgender, non-binary, and intersex communities.
Sexuality
Speaking of dangerous AI systems that have no possible positive use case: Gaydar is among the most offensive ideas in the machine learning world.
Artificial intelligence cannot predict a person’s sexuality because, you guessed it: psychics don’t exist. Humans cannot tell if other humans are gay or straight unless the subject of scrutiny expressly indicates exactly what their sexuality is.
[Read: The Stanford Gaydar is hogwash]
Despite the insistence of various members of the I’m-straight and I’m-gay crowds, human sexuality is far more complex than whether or not we’re born with gay face because our moms gave us different hormones, or if we’re adverse to heterosexual sexual encounters because… whatever it is that straight people think makes gay people gay these days.
In the year 2020 some scientists are still debating whether bisexual men exist. As an out pansexual, I can’t help but wonder if they’ll be debating my existence in another 20 or 30 years when they catch up to the fact that “gay and straight” as binary concepts have been outdated in the field of human psychology and sexuality since the 1950s. But I digress.
You cannot build a machine that predicts human sexuality because human sexuality is a social construct. Here’s how you can come to that same conclusion on your own:
Imagine a 30 year old person who has never had sex or been romantically attracted to anyone. Now imagine they fantasize about sex with women. A day later they have sex with a man. Now they fantasize about men. A day later they have sex with a woman. Now they fantasize about both. After a month, they haven’t had sex again and stop fantasizing. They never have sex again or feel romantically inclined towards another person. Are they gay, straight, or bisexual? Asexual? Pansexual?
That’s not up to you or any robot to decide. Does thinking about sex account for any part of your sexuality? Or are you “straight until you do some gay stuff?” How much gay stuff does someone have to do before they get to be gay? If you stop doing gay stuff can you ever be straight again?”
The very idea that a computer science expert is going to write an algorithm that can solve this for anyone is ludicrous. And it’s dangerous.
There is no conceivable good that can come from Gaydar AI. It’s only use is as a tool for discrimination.
Intelligence
AI cannot determine how intelligent a person is. I’m going to flip the script here because this has nothing to do with being psychic. When AI attempts to predict human intelligence it’s performing prestidigitation. It’s doing a magic trick and, like any good illusion, there’s no actual substance to it.
We can’t know a person’s intelligence unless we test it and, even then, there’s no universally recognized method of measuring pure human intelligence. Tests can be biased, experts dispute which questions are best, and nobody knows how to deal with hyperintelligent humans with mental disorders. Figuring out how smart a person is can’t be solved by a few algorithms.
So what do these AI systems do? They search for evidence of intelligence by comparing whatever data they’re given on a person to whatever model for intelligence the developers have come up with. For instance, they might determine that an intelligent person doesn’t use profanity as often as a non-intelligent person. In this instance, Dane Cook would be considered more intelligent than George Carlin.
That’s a comedic way of looking at it, but the truth is that there’s no positive use case for a robot that arbitrarily declares one human smarter than another. But there are plenty of ways these systems can be used to discriminate.
Potential
Ah yes, human potential. Here I want to focus on hiring algorithms, but this applies to any AI system designed to determine which humans, out of a pool, are more likely to succeed at a task, job, duty, or position than others.
Most major companies, in some form or another, use AI in their hiring process. These systems are almost always biased, discriminatory, and unethical. In the rare cases they aren’t, it’s where they seek out a specific, expressed, qualification.
If you design an AI to crawl thousands of job applications for “those who meet the minimum requirement of a college degree in computer science” with no other parameters… well, you could have done it quicker and cheaper with a non-AI system… but I guess that wouldn’t be discriminatory.
Otherwise, there’s no merit to developing AI hiring systems. Any data they’re trained on is either biased or useless. If you use data based on past successful applicants or industry-wide successful applicants, you’re entrenching the status quo and intentionally avoiding diversity.
The worst systems however, are the ones purported to measure a candidate’s “emotional intelligence” or “how good a fit” they’ll be. AI systems that parse applications and resumes for “positive” keywords and “negative” keywords as well as video systems that use “emotional recognition” to determine the best candidates are all inherently biased, and almost all of them are racist, sexist, ageist, and ableist.
AI cannot determine the best human candidate for a job, because people aren’t static concepts. You can’t send a human or a machine down to the store to buy a perfect HR fit. What these systems do is remind everyone that, traditionally, heterosexual, healthy, white men under the age of 55 is what most companies in the US and Europe hire, so it’s considered a safe bet to just keep doing that.
And there you have it, six incredibly popular areas of AI development – I’d estimate that there are hundreds of startups working on predictive policing and hiring algorithms alone – that should be placed on any ethical developer’s do not develop list.
Not because they could be used for evil, but because they cannot be used for good. Each of these six AI paradigms are united by subterfuge. They purport to solve an unsolvable problem with artificial intelligence and then deliver a solution that’s nothing more than alchemy.
Furthermore, in all six categories the binding factor is that they’re measured by an arbitrary percentage that some how indicates how “close” they are to “human level.” But “human level” in every single one of these six domains, means “our best guess.”
Our best guess is never good enough when the “problem” we’re solving is whether a specific human should be employed, free, or alive. It’s beyond the pale that anyone would develop an algorithm that served to only bypass human responsibility for a decision a robot is incapable of making ethically.
Published July 31, 2020 — 19:37 UTC
August 01, 2020 at 02:37AM
https://ift.tt/2BSIxXq
The 6 unholy AI systems thou shalt not develop - The Next Web
https://ift.tt/2APQ0pp
MIT’s machine learning designed a COVID-19 vaccine that could cover a lot more people - ZDNet
There are currently 25 vaccines to fight COVID-19 in clinical evaluation, another 139 vaccines in a pre-clinical stage, and many more being researched.
But many of those vaccines, if they are at all successful, might not produce an immune response in portions of the population. That's because some people's bodies will react differently to the materials in the vaccine that are supposed to stimulate virus-fighting T cells.
And so just figuring out how much coverage a vaccine has, meaning, how many people it will stimulate to mount an immune response, is a big part of the vaccine puzzle.
With that challenge in mind, scientists at Massachusetts Institute of Technology on Monday unveiled a machine learning approach that can predict the probability that a particular vaccine design will reach a certain proportion of the population. That doesn't mean they can guarantee its effectiveness, but the scientists' work can aid in knowing up-front whether a given vaccine will have large gaps in who it can help.
The good news is, the MIT scholars have used their approach to design a novel COVID-19 vaccine on the computer that has far better coverage than many of the designs that have been published in the literature this year. They're now testing the design in animals.
The bad news is, there could very well be large gaps in coverage of some of the existing vaccines already being explored by companies and labs, according to one of the authors of the report, David K. Gifford, who is with MIT's Computer Science and Artificial Intelligence Laboratory.
"While they may protect more than 50% of the population, certain individuals and older individuals may not be protected," Gifford told ZDNet in an email, when asked about vaccines currently under trial and in development.
The long path to a vaccine
Vaccines in development were not the direct subject of the work. Most of those vaccines are closed designs; no one knows exactly how they are composed. Instead, Gifford and colleagues designed vaccines from scratch, and then analyzed how effective they are, and extrapolated the findings to a group of vaccines whose composition is known.
Based on that, one can infer there might be problems with vaccines whose exact composition is not known.
It must be borne in mind that any in silico vaccine design such as the kind discussed here is only the beginning of a process that can take years to go through in vivo testing, in animals and then in humans, to establish both safety (non-toxicity), and efficacy, meaning that it actually confers a significant immune response.
Also: MIT's deep learning found an antibiotic for a germ nothing else could kill
But the work shows the ability of large computer models to dramatically speed up the initial work of searching through many, many possible combinations within a universe of possible ingredients, a search that can itself take years at the front end of a drug development pipeline.
This is the latest in large-scale, in-silico efforts against pathogens seen this year from MIT. Back in March, ZDNet reported on how MIT scientists used large-scale machine learning to search many combinations of compounds to come up with a novel antibiotic for a germ nothing else could kill.
A combinatorial challenge
The present work, titled, "Computationally Optimized SARS-CoV-2 MHC Class I and II Vaccine Formulations Predicted to Target Human Haplotype Distributions," is published in Cell Systems, an imprint of Cell Press, part of Elsevier. Authors include Gifford as the corresponding author; lead authors Ge Liu and Brandon Carter of the AI lab; Trenton Bricken of Duke University; Siddhartha Jain, also of the AI lab; and Mathias Viard and Mary Carrington, who have dual roles at Mass General and at the Frederick National Laboratory for Cancer Research in Maryland. (A blog post has also been provided by MIT.)
Gifford and colleagues built a program that designs a vaccine based on two different criteria, the intersection of which is a combinatorial problem.
The first criterion is whether parts of a virus bind to proteins on the surface of a human cell. The bits of virus, which are short strings of perhaps 8 to 25 amino acids, are known as peptides. The human proteins are what are known as surface cell receptors.
When an invading organism enters the body, such as a virus, some of the peptides of that organism fit into a groove in the surface cell receptor. The surface cell receptor then presents that peptide to the body's T cells as a signal of the invasion. The T cells begin a process of killing off such infected cells. That's how natural human immunity works.
But humans need help sometimes, they need to be primed to respond, and that's what vaccines do. Vaccines duplicate this natural immune process before someone is infected, to get the body prepped to generate a T-cell response. To figure out which peptides will fit in the groove of surface cell receptors is a matching problem on a large scale: which of thousands of peptides go with thousands of variants of different surface cell receptors the body can produce.
That's part one. The second part of the problem is asking which people in the population have the combination of alleles, genetic variants of the surface cell receptor, that will work with a certain group of peptides. It's a matter of finding the lowest common denominator in the matching search, which peptide-receptor combinations are common among the greatest number of individuals.

A diagram of the workflow of MIT's machine learning programs for vaccine design. The OptiVax algorithm searches for optimal binding pairs of peptides and human cell surface receptor proteins. It is composed of a novel assembly of eleven existing machine learning search programs. Its objective function is the information about optimal population coverage fed to it by the second algorithm, EvalVax, which analyzes frequency of genetic variants across the population. Two versions of each program options in the workflow, a simpler version called Unliked and a more sophisticated version, known as Robust, which tracks not just single variants in human genes but linked sets of variants known as haplotypes. The option to cover haplotypes is an advanced feature that sets the search apart from past efforts.
Liu et al. 2020An exhaustive search
To achieve both results, the researchers built two machine learning programs. One, called OptiVax, performs the matching search on a scale never achieved before. It combines eleven different pre-existing programs designed to test combinations of peptides and receptors, an ensemble, as Gifford and colleagues call it.
"This is to our knowledge the first application of combinatorial optimization to peptide vaccine design," Gifford told ZDNet, "and it is a challenging computational task that required an efficient implementation."
Just identifying the relevant peptides, about 155,000 in this case, was the first challenge, breaking down the SARS-CoV-2 genetic sequence into its components. Then OptiVax had to go to work on choosing amongst them to pick the best handful, or set, of peptides on which to focus.
"Previous work did not do this exhaustive search," Gifford told ZDNet.
A second program, called EvalVax, takes population data from thousands of individuals who self-reported across three categories, white, Black, and Asian. You could call these ethnicities, and that term is used in the report. Another term that has been proposed in prior work is genetic ancestry. In a 2015 paper, Tesfaye B. Mersha and Tilahun Abebe of the University of Cincinnati proposed ancestry as a better term for genetic distinctions in groups of the population, versus ethnicity, which has more to do with "traditions, lifestyle, diet, and values," they wrote.
A new machine
The two programs, OptiVax and EvalVax, work in tandem in a feedback loop. More specifically, the population program EvalVax, which knows how common alleles are in the three groups of ancestry, serves as the objective function to the search that OptiVax is conducting over peptide-receptor pairs.
All that translates into "about 12 hours on a large multiprocessor computer to design one vaccine using our methods," said Gifford.
OptiVax's ensemble is itself the result of years of prior work by other researchers to create machine learning-based peptide screens. One of the most prominent software programs is called NetMHCPan, developed in 2007 by Morten Nielsen and colleagues at the Technical University of Denmark. NetMHCPan uses a feed-forward neural network. The network is fed pairs of peptide and receptor as its input data, and it generates a predicted binding, or affinity, score, as its output. That score is tested against known bindings that have already been established experimentally, as the supervised training step.
The network's binding predictions are then improved with repeated attempts, via the back-propagation method. Over the years, the program has gone through several revisions and is available as a Web-based server and for download.
The latest in deep learning techniques
The OptiVax program that Gifford and colleagues developed combines NetMHCPan's predictions with predictions from similar screening programs. To get a consensus from the ensemble, OptiVax uses a technique called beam search, which has become ubiquitous in natural language programs. It forms the decoder in software such as Google's BERT and OpenAI's GPT-3. Beam search evaluates a host of possible combinations of elements to find the most likely combo.
To create EvalVax, the objective function that measures population coverage, Gifford and team went beyond the past attempts to measure population coverage. Such studies only asked how common a given genetic variant might be, the allele. But some alleles' frequency can be connected to how common or rare other alleles are, a phenomenon known as linkage disequilibrium.
For that reason, it can be important to look at how common whole combinations of alleles are, known as haplotypes. That, again, brings a combinatorial challenge that is larger, more complex. The approach, however, is a better way to design vaccines, insists Gifford.
"Unlike previous methods, we use HLA haplotype frequencies to score and design vaccines which is a more accurate way of predicting vaccine coverage than the previous use of independent HLA frequencies," said Gifford. HLAs is the technical term for the cell surface receptors that bind with the peptides.
A better vaccine
The result of all this is that OptiVax came up with some vaccine designs consisting of peptide-receptor pairs that have better coverage than designs other teams have come up with since the pandemic started. In what appears reminiscent of many machine learning benchmark tests, the authors report how the coverage of their recipe of peptides compares versus what they estimate to be coverage for the many vaccine proposals in the literature.
In one instance, OptiVax came up with a collection of 19 peptides that would have a 99.91% probability of at least one of the peptides binding to any haplotype of a person in any of the three ancestry groups. That percentage probability of at least one hit was well above the percentage probability for at least one hit in the other vaccine proposals they surveyed from the literature.
As they write in the paper, "We observed superior performance of OptiVax-Robust-designed vaccines on all evaluation metrics at all vaccine sizes […] Most baselines achieved reasonable coverage […] However, many failed to show a high probability of higher hit counts."
A separate question from coverage is how much immunity is conferred by a given vaccine design. With a novel disease like COVID-19, scientists are still finding out which immune responses are neutralizing, meaning, able to retard or stop completely the functioning of the virus.
Happily, there is evidence that peptides that successfully bind to a receptor have a better chance of producing the neutralizing response.
"When peptides do bind to class I MHC molecules, it has been shown in mouse models that almost all binding peptides are immunogenic," Gifford told ZDNet. He was referring to to the major histocompatibility complex, the area of the human genome that produces the receptors. A study in 2018 by Washington University researchers, Gifford noted, found that "a surprisingly high fraction" of such peptides produced a neutralizing response, what's known as immunogenicity.
On the contrary, Gifford cautions that many drugs in development may miss the mark in neutralization even if they stimulate some response.
"While it is early days, clinical study data on candidate vaccines that has been released has shown that not all individuals develop a robust cellular immune response to COVID-19." Gifford speculates that, as EvalVax suggests, those vaccines "have population coverage gaps in peptide binding," which, he said, "could influence durability and response in older individuals."
A complicating factor is that COVID-19 continues to evolve genetically, so that some proteins change over time, making it harder to target the peptides they contain.
The mutation rate appears to be small enough at present not to be a substantial issue, Gifford told ZDNet.
"We can not guarantee that there will not be further viral sequence drift," said Gifford. "However, the lack of, or low rate of mutation of our candidate peptides over our more than 4,000 geographically-sampled genomes suggests that these peptides may be functionally required and thus less likely to drift in the future."
Ancestries challenge coverage
One nagging problem persists despite the significant improvement observed in OptiVax's design: even with better overall coverage, some of the results are mixed according to ancestry.
When comparing vaccine designs from OptiVax in terms of having two or more peptide hits, the percentage probability declines for all three ancestry groups, but it declines unevenly. The likelihood of having multiple hits starts to show great disparity at five or more hits, with those of Asian ancestry showing the greatest likelihood of the full number of hits, those of white ancestry showing somewhat less, and those self-identifying as of Black ancestry showing less of a chance than either two.
That's important, because any one peptide-receptor hit might not turn out to be effective in a given individual, so it's better, if possible, to have multiple potential peptides to increase the odds a drug works on a given individual.
It's tempting to think that adjusting the search techniques could reduce that disparity. In the present paper, Gifford and colleagues focused on what's called precision, meaning, making sure that there are as few as possible false positives in their peptide selections. Because drugs can take a long time to develop, a lot of effort can be wasted if early positive indicators later turn out to have been misleading.
Also: How a smartphone coupled with machine learning may become a simple, efficient test for COVID-19
But focusing on precision in this case meant less emphasis on what's called recall, which is the number of true positives found out of all the true positives that exist in a universe of possibilities. It would be nice to think that adding more focus to recall could lead to more peptides that would bind across the haplotypes of all three ancestries, or at least, more evenly so.
That may not be the case, however.
"Our estimates of self-reporting ancestry-based haplotype populations suggests that certain ancestries may be intrinsically harder to cover," Gifford told ZDNet when asked about increasing recall. In fact, greater recall could be misleading, as it "would make the numbers look better, but it would potentially overestimate population coverage and as a consequence provide less robust vaccine designs."
Gifford cautions not to read too much into the disparities at high hit counts, as those disparities can be a result of "many factors."
Gauging COVID-19's risk
In the meantime, there is ongoing work to take the OptiVax design to the next level. "We are working with both academic and commercial collaborators to test OptiVax-derived designs in animal models," Gifford told ZDNet. "If the designs show promise in these models, the next logical step would be clinical trials."
Beyond just drug development, this kind of combinatorial analysis can pay a lot of other dividends. A separate project of the authors is currently underway examining the blood sera of people who have recovered from COVID-19 to gauge how much immunity those individuals developed.
One might wonder, too, if the OptiVax and EvalVax findings reveal anything about the pathogenesis of COVID-19. Is there anything that can be said about the different ancestries' peptide binding rates that reflects upon those populations' response to the disease?
It turns out that Gifford and team have also added that question to their work. They are comparing the patterns of peptide and receptor matches they have found to patterns of COVID-19 severity in patients, accompanied by analysis of healthy control subjects, to do risk analysis of the disease, Gifford told ZDNet.
Vaccine makers should open up
The authors have some choice words for those developing vaccines. Consistent with the warning that Gifford gave ZDNet in email, the concluding section of the published paper notes a big potential problem with many vaccines. They tend to focus a lot on the most notorious protein of the SARS-CoV-2 virus, called the S, or spike, protein. That is because biological analysis suggests the S protein should produce antibodies that are of the neutralizing kind.
But the OptiVax test suggests the S protein may not have complete coverage of the population. "Vaccines that only employ the S protein may require additional peptide components for reliable CD4+ T cell activation across the entire population," the authors write. They suggest ways of adding peptides to S-based drugs to enhance coverage.
On a deeper note, Gifford and team urge drug developers to put their designs out in the open to be scrutinized. "The precise designs of most of these vaccines are not public," they note. "We encourage the early publication of vaccine designs to enable collaboration and rapid progress toward safe and effective vaccines for COVID-19."
The OptiVax code and data sets of its peptide predictions are available on Github.
Coronavirus
July 31, 2020 at 08:45PM
https://ift.tt/2DaQ2cF
MIT’s machine learning designed a COVID-19 vaccine that could cover a lot more people - ZDNet
https://ift.tt/2APQ0pp
Featured Post
Latest iPhone 13 Dummies Again Show New Diagonal Dual-Lens Camera Arrangement Coming to Standard Model - MacRumors
doro.indah.link More dummy models of the upcoming iPhone 13 have been shared online, this time by leaker DuanRui , indicating the new diag...
/article-new/2021/06/iphone-13-duan-rui2.jpeg?lossy)
Popular Posts
-
doro.indah.link Samsung’s Good Lock suite of customization tools recently received two exciting new modules — Pentastic and Wonderland. I...
-
doro.indah.link ILMxLAB is again teaming up with VR arcade company Nomadic to bring a new version of the ‘Lightsaber Dojo’ from Vader Immo...
-
doro.indah.link More dummy models of the upcoming iPhone 13 have been shared online, this time by leaker DuanRui , indicating the new diag...